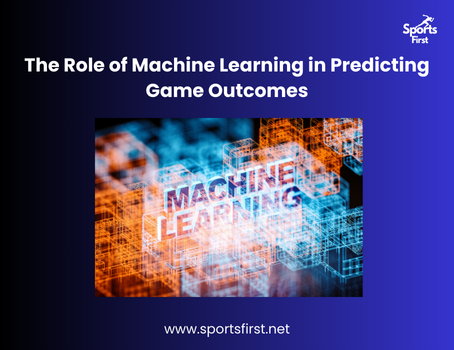
In the ever-evolving landscape of sports and gaming, the ability to predict game outcomes has long been a fascinating challenge. From casual fans to seasoned bettors and professional analysts, everyone is interested in making accurate predictions. With the advent of machine learning (ML), the process of predicting game outcomes has become more sophisticated, data-driven, and precise. Machine learning leverages vast amounts of historical data, statistical models, and advanced algorithms to make predictions that often surpass human intuition.
This article explores the role of machine learning in predicting game outcomes, including the methodologies, advantages, limitations, and real-world applications of this technology.
How Machine Learning Works in Game Predictions
Machine learning is a subset of artificial intelligence (AI) that enables computers to learn from data and make predictions or decisions without being explicitly programmed. When applied to predicting game outcomes, ML models analyze a vast range of factors, such as team statistics, player performance, weather conditions, historical results, and even social media sentiment.
1. Data Collection and Processing
The first step in any ML-based prediction model is data collection. Relevant datasets include:
Team and Player Statistics: Win/loss records, average points per game, shooting percentages, injury reports, and more.
Game Situations: Home/away games, team form, fatigue levels, and travel schedules.
External Factors: Weather conditions for outdoor sports, referee biases, and even crowd influences.
Sentiment Analysis: Fan reactions and expert opinions from social media and sports analysts.
Once collected, the data is cleaned, normalized, and processed to remove inconsistencies and ensure high-quality inputs for the model.
2. Feature Engineering
Feature engineering involves selecting and transforming variables that have the highest impact on game outcomes. Some key features might include:
Recent form trends (last five games performance)
Head-to-head matchups
Key player availability
Betting odds fluctuations
Advanced metrics like expected goals (xG) in soccer
3. Model Selection and Training
Various machine learning models are used to predict game outcomes, including:
Logistic Regression: A simple yet effective classification algorithm for predicting win/loss outcomes.
Decision Trees & Random Forests: These models help analyze different game scenarios and their impact on results.
Neural Networks: Deep learning models that analyze complex, non-linear patterns in large datasets.
Bayesian Networks: Probabilistic models that update predictions as new information becomes available.
Reinforcement Learning: Algorithms that learn optimal strategies through trial and error, particularly useful in esports and gaming AI.
The chosen model is trained using historical data, and once it achieves acceptable accuracy levels, it is deployed for real-time predictions.
Applications of Machine Learning in Game Predictions
1. Sports Betting and Fantasy Sports
One of the biggest industries benefiting from ML-driven game predictions is sports betting. Algorithms analyze odds and historical data to identify value bets and recommend profitable wagers. Similarly, fantasy sports platforms use ML to suggest optimal lineups based on player projections.
2. Team Strategy and Performance Analysis
Professional teams use machine learning to analyze opponents’ strategies and optimize their own game plans. By studying past performances and tactical patterns, coaches and analysts can make data-driven decisions on formations, substitutions, and defensive strategies.
3. Broadcasting and Fan Engagement
Sports broadcasters use ML to generate real-time predictions and insights for viewers. AI-driven analysis enhances commentary, offers interactive dashboards, and provides fans with deeper insights into the game’s potential outcomes.
4. Esports and Video Games
In the gaming industry, ML algorithms predict in-game events, optimize matchmaking, and even design adaptive AI opponents. Esports analysts use machine learning to forecast match results and suggest optimal team compositions.
Advantages of Machine Learning in Game Predictions
1. Data-Driven Accuracy
ML models process thousands of variables simultaneously, offering more accurate predictions than human analysts relying on intuition.
2. Real-Time Adaptability
Unlike traditional statistical models, ML algorithms continuously learn and improve over time as they ingest new data.
3. Bias Reduction
Human biases often cloud judgment in predicting game outcomes. ML algorithms, when properly trained, minimize these biases by relying on empirical data.
4. Scalability
Once an ML model is trained, it can analyze vast amounts of data in seconds, making it scalable for large-scale applications like sports betting platforms.
Limitations and Challenges
1. Data Quality and Availability
The accuracy of an ML model depends on the quality of the data fed into it. Incomplete or biased data can lead to misleading predictions.
2. Unpredictability in Sports
Sports inherently involve elements of randomness—unexpected injuries, referee decisions, or clutch performances—that ML models struggle to account for.
3. Overfitting
If an ML model is too complex, it may overfit to historical data and fail to generalize to new games, reducing its predictive accuracy.
4. Ethical Concerns
The use of ML in sports betting raises ethical questions about fairness, transparency, and the potential for insider advantages.
Future of Machine Learning in Game Predictions
The future of ML in game predictions is promising, with advancements in deep learning and AI expected to enhance accuracy further. Predictive analytics will become more refined with the integration of real-time biometric data, player emotions, and AI-driven game simulations. Additionally, hybrid models combining machine learning with expert human analysis will offer even more reliable predictions.
As ML continues to evolve, it will not only transform sports betting but also revolutionize team strategies, broadcasting, and the overall fan experience. The intersection of AI and sports analytics is just beginning, and the possibilities are endless.
Machine learning is rapidly transforming the way game outcomes are predicted, offering unparalleled accuracy, scalability, and adaptability. From sports betting to esports and professional team analysis, ML-driven predictions are becoming an integral part of the gaming ecosystem. While challenges like data quality and unpredictability remain, continuous advancements in AI and deep learning promise an exciting future where game predictions are more precise than ever before.
As technology progresses, machine learning will not replace human intuition but rather complement it, providing deeper insights and revolutionizing the way we engage with sports and gaming. Whether you are a bettor, a fan, or a team strategist, embracing ML-driven predictions could be the key to staying ahead of the competition.